9 Must-Know Technology Terms for Finance and Technology Professionals
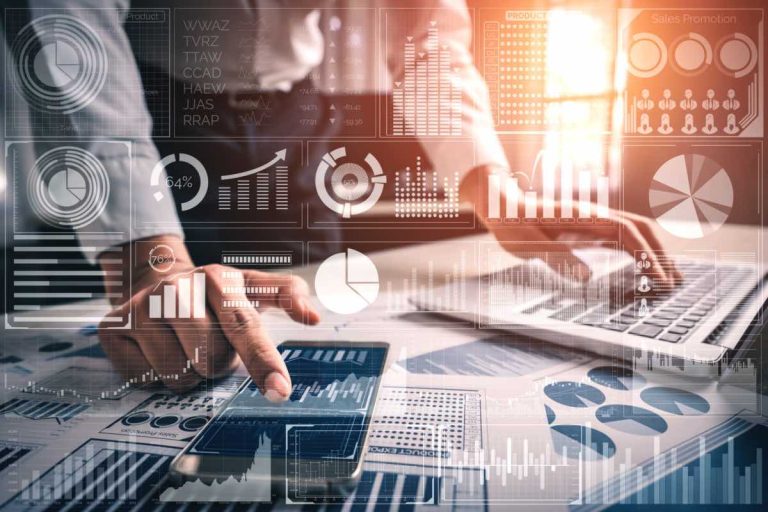
As businesses continue to adapt and move forward, corporate functions are becoming more and more intertwined. Historically speaking, we’ve seen functions like IT and Finance operating solely on their own, selfishly tackling objectives geared to their interests and goals. With the merging of technologies, organizations are breaking down silos to leverage an integrated approach to drive forward.
Data and Analytics have been a hot topic over the years that could arguably be a primary reason for merging departments. With so much data readily available for organizations to digest, it’s been an industry that’s exploded in growth. Yet, it demands a seamless integration between all processes, workstreams, and unanimous adoption and understanding of industry terminology to be effective. DLC, a financial consulting firm, regularly works with Data and Analytics teams, finding ourselves continuously learning new terminology and processes.
If you’ve ever found yourself speaking with a data scientist, you may have already experienced the lingo and almost foreign language that they speak in. Today, we’ll cover 9 of the most common vocabulary we run across during our engagements.
1. Agile
Agile is a project management philosophy used to acknowledge that project scope is always changing. Instead of waiting for a final presentation to the conclusions, we remain in constant contact with the customer and team members to share working versions of the end-product through the iterative cycles. An agile methodology greatly minimizes negative surprise factors while allowing for flexibility as the project scope changes.
2. Scrum
Scrum is a derivative of Agile that is more intense and more defined. Team members set goals for brief periods and frequently meet to discuss, gauge progress, and update and change based on feedback.
3. Industry 4.0 / Digital Manufacturing
Traditional manufacturing/supply chain is transformed using advanced computing power, interconnectivity, and machine learning/AI. The ongoing automation of conventional practices and widespread adoption of modern technology can be thought of as “the Fourth Industrial Revolution” within the manufacturing industry. Visibility, transparency, and enhanced data allow manufacturing and supply chain teams to have prompt feedback, better anticipate changes in demand and plan.
4. Lean Manufacturing
Lean manufacturing is relatively simple to understand – minimize waste, maximize productivity. Operations teams are continually looking for ways to improve existing processes by identifying waste and removing steps that ultimately do not add value to the end customer. The operations focus begins with customer demand and ends with customer satisfaction.
5. Zero-Based Budgeting
Zero-based budgeting turns traditional budgeting and forecasting on its head. Unlike traditional budgeting, start from 0 for every line in the budget and provide justifications for each dollar spent. Legacy costs and blanket increments are thrown out of the window. Zero-Based budgeting could be a resource-intensive method, but it is recommended that this method be followed once every couple of years to ensure a fresh and current perspective and eliminate wasteful spending.
6. Product Teardowns/Clean Sheet
As products evolve and develop, it’s crucial to analyze what’s being used, why, and the associated costs. A product teardown helps accomplish this by conducting a deep dive into product cost structure and a thorough analysis of what costs are incurred, why, and how they are incurred. Once this analysis has been completed, organizations will often engage in product cost optimization exercises.
7. Machine Learning
Machine learning is an application of artificial intelligence to enable computer programs/algorithms to learn insights from data independently. Successful machine learning relies upon the use of clean data, guidelines, and direction. The underlying statistical methods are already built into software products in the market. The skillset available is mostly focused on how and when to employ automation. Machine learning is used across various facets of technology, from news feeds to Netflix recommendations and everything in between.
8. Deep Learning
Deep Learning is an advanced version of machine learning where algorithms learn what to learn and learn independently. Minimum supervision or direction is required as results are automatically validated. Algorithms are optimized over time and use neural networks like structures built to break problems into many sub-components and find solutions. One example of deep learning is autonomous driving.
9. Random Forest/Regression Tree
Random Forst/Regression Tree has nothing to do with a real forest. Instead, this is a predictive tool that builds decision trees to classify inputs to generate an output. The key is to have different types of trees (input classes) to predict overall prediction accuracy better. An example of a regression tree involves marketing analysis. The more types of customers exist for us to target, the better our comprehensive ad targeting will be.
Putting it all together
As a finance professional working in technology, there is endless terminology. Be sure to stay up to date with tech and best practices to help ensure your success. DLC’s dedicated team of consulting professionals is here to help you navigate this dynamic landscape, from revamping your business strategy to executing essential finance and cash management initiatives.
Check out our full suite of services here. Ready for a solution? Need some guidance? Let’s have a conversation.
About the Author:
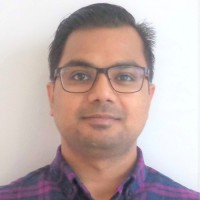
Nil Swain is an MBA/PMP consultant in DLC’s Chicago office. Nil has consulted with the firm for the last seven years, focusing on leadership and execution roles supporting the middle market and Fortune 500 companies with financial planning & analysis, inventory, supply chain optimization, ERP, and financial system implementations.